The Assignment
A mid-level investing bank needed to implement a stress testing framework in accordance with guidelines issued by the central bank.
Our mandate was to develop a collaborative, auditable, repeatable, and transparent stress testing program to meet regulatory expectations, inform on the bank’s risk appetite framework, and improve strategic business decisions.
Our Solution
The solution included the following key features:
1. Stress testing
Our stress testing framework comprised of regular stress tests and scenario analysis with severe macroeconomic global downturn scenarios. We included all material risk types into our stress testing exercises which included portfolio- and country-specific stress tests.
2. ICAAP
Capital plan stress testing was performed to assess the viability of the capital plan in adverse circumstances and to demonstrate a clear link between risk appetite, business strategy, capital plan and stress testing.
3. Scenario Analysis
The stress test framework also consisted of defined macroeconomic downturn scenarios which were based on quantitative models and expert judgments, economic parameters such as foreign exchange rates, interest rates, GDP growth or unemployment rates.
4. Reverse Stress Testing
Reverse stress test were to be performed annually in order to determine the severity of scenarios that would cause the bank to become unviable.
5. Risk Reporting and Measurement
The stress testing framework supported regulatory reporting and external disclosures, as well as internal management reporting, to be presented to senior management as well as to the risk committees, who are responsible for risk and capital management.
Key Takeaways for the Client
A robust stress testing framework that integrated governance, documentation, data quality management, economic scenario development, loss modeling, forecasting, and reporting and incorporated participation of all stakeholders across business units.
The framework was integrated across asset classes and lines of business and enhanced risk management and was customized to the unique strategies and risks of the bank’s portfolio
INTERNATIONAL PARTNERS
NEW YORK & INDIA

Our work in developing a Credit Scoring model
The Assignment
Our client, a Non-Banking Finance Corporation that specializes in lending to Small and Medium retail enterprises, needed to create a credit model based on predictive analytics to make lending decisions for customers with limited credit history. The borrower’s eligibility and sanction of loan needed to be guided by assessment of character, credit worthiness, capital, willingness and capacity to repay loan using the proprietary credit scoring model.
Our Solution
The client was aiming to service the small and medium enterprises who are unable to avail credit from conventional lenders such as commercial banks and finance companies. Small business development in India is characterized by difficult access to the capital, legal and law limitations, undeveloped infrastructure, high transactional costs, as well as high loan interest rates.
As the large number of small and medium retail businesses utilize credit from what is called the shadow banking industry, conventional methods of credit scoring are not effective because of lack of financial data, non-existence of a formal credit history etc. We needed to develop a new credit scoring model based on logical regression which would be based on information about the borrower, allowing us to differentiate between the “good” and the “bad” loans and determine a probability of default.
Our predictive model learnt by utilizing the customers’ historical data together with peer group data from his/her own sector as well as other data to identify ‘good’ applications and ‘bad’ applications on an individual basis, and also forecast the probability that an application with any given score will be ‘good’ or ‘bad’. These probabilities or scores, along with other business considerations, such as profit, churn, and losses, were then used as a basis for decision making for the loan as well as the amount of money to be loaned to the customer, the interest rate that should be charged and the repayment terms of the loan.
Key Takeaways for the Client
With the automation of the loan process, decisions on loans could be made almost instantly cutting down the processing time for each loan. By using unconventional data, the client was able to safely extend credit to clients who are deemed too ‘risky’ using conventional credit scoring methods.
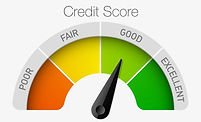